Inverse Design for Materials Discovery from the Multidimensional Electronic Density of States
-
Authors :
Kihoon Bang, Jeongrae Kim, Doosun Hong, Donghun Kim, Sang Soo Han
-
Journal :
Journal of Materials Chemistry A
-
Vol :
12
-
Page :
6004-6013
-
Year :
2024
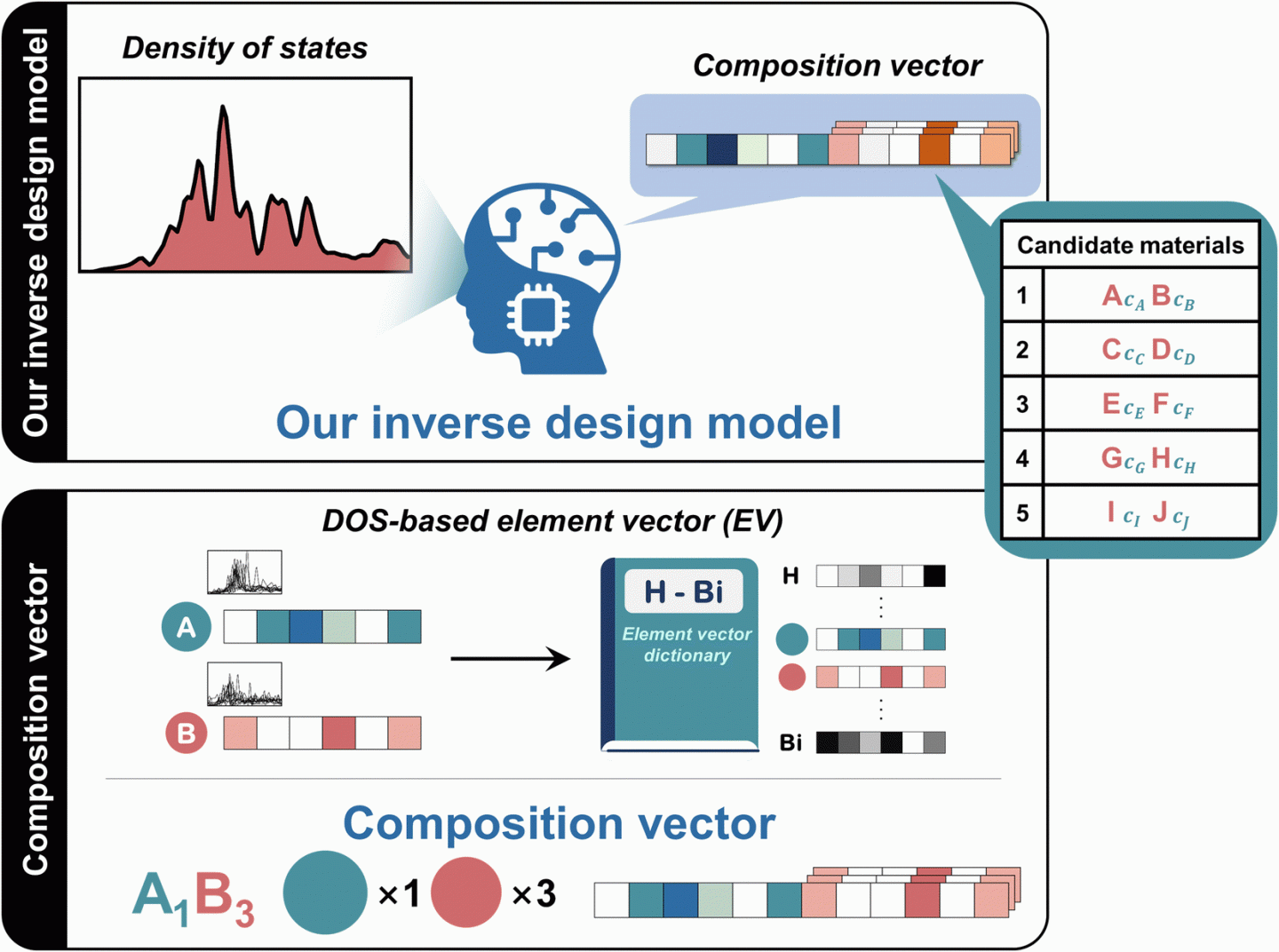
Abstract
To accelerate materials discovery, an inverse design scheme to find materials with desired properties has been recently introduced. Despite successful efforts, previous inverse design methods have focused on problems in which the desired properties are described by a single number (one-dimensional vector), such as the formation energy and bandgap. The limitation becomes apparent when dealing with material properties that require representation with multidimensional vectors, such as the electronic density of states (DOS) pattern. Here, we develop a deep learning method for inverse design from multidimensional DOS properties. In particular, we introduce a composition vector (CV) to describe the composition of predicted materials, which serves as an invertible representation for the DOS pattern. Our inverse design model exhibits exceptional prediction performance, with a composition accuracy of 99% and a DOS pattern accuracy of 85%, greatly surpassing the capabilities of existing CVs. Furthermore, we have successfully applied the inverse design model to find promising candidates for catalysis and hydrogen storage. Notably, our model suggests a hydrogen storage material, Mo3Co, that has not yet been reported. This readily reveals that our model can greatly expand the space of inverse design for materials discovery.