Machine learning-enabled exploration of the electrochemical stability of real-scale metallic nanoparticles
-
Authors :
Kihoon Bang, Doosun Hong, Youngtae Park, Donghun Kim, Sang Soo Han, Hyuck Mo Lee
-
Journal :
Nature Communications
-
Vol :
14
-
Page :
3004
-
Year :
2023
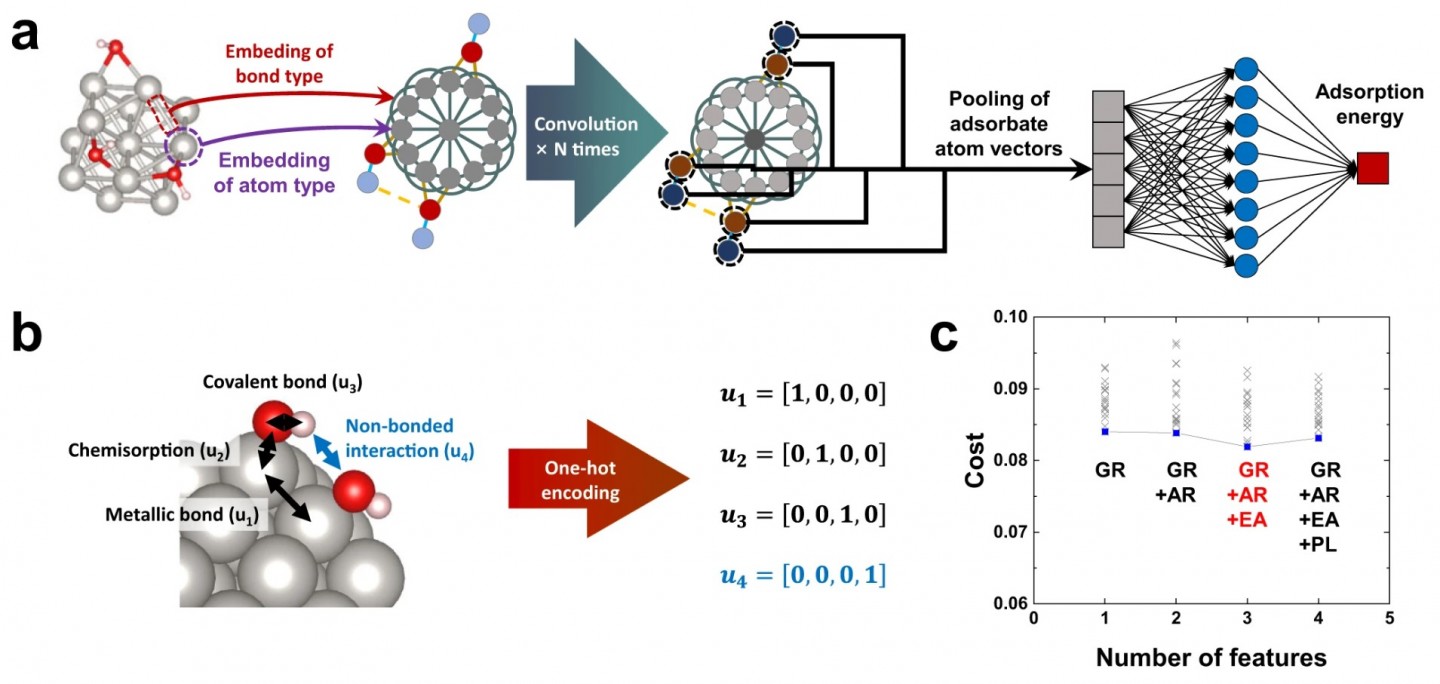
Abstract
Surface Pourbaix diagrams are critical to understanding the stability of nanomaterials in electrochemical environments. Their construction based on density functional theory is, however, prohibitively expensive for real-scale systems, such as several nanometer-size nanoparticles (NPs). Herein, with the aim of accelerating the accurate prediction of adsorption energies, we developed a bond-type embedded crystal graph convolutional neural network (BE-CGCNN) model in which four bonding types were treated differently. Owing to the enhanced accuracy of the bond-type embedding approach, we demonstrate the construction of reliable Pourbaix diagrams for very large-size NPs involving up to 6525 atoms (approximately 4.8 nm in diameter), which enables the exploration of electrochemical stability over various NP sizes and shapes. BE-CGCNN-based Pourbaix diagrams well reproduce the experimental observations with increasing NP size. This work suggests a method for accelerated Pourbaix diagram construction for real-scale and arbitrarily shaped NPs, which would significantly open up an avenue for electrochemical stability studies.